A CISO’s Guide to the AI Opportunity in Security Operations
The artificial intelligence (AI) frenzy is in full swing. Across all sectors, business leaders and decision makers are scrutinizing potential use cases for this technology, envisioning new ways to empower their workforce and streamline processes. Yet, in this surge of enthusiasm strategists simultaneously need to be aware of the novel issues, concerns, and threats AI may introduce.
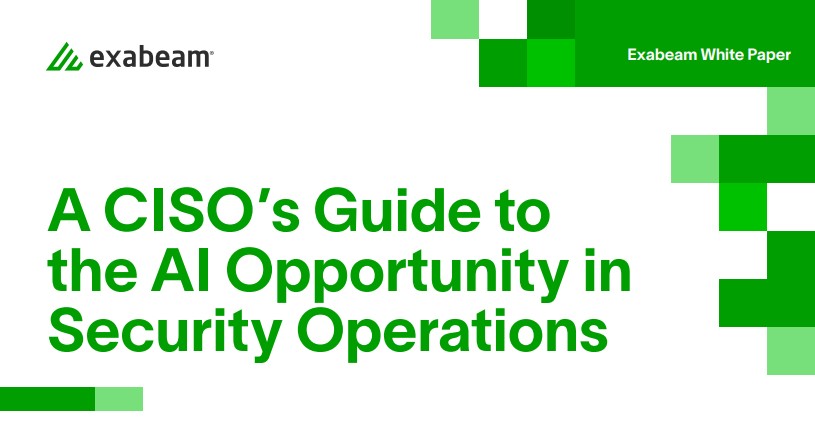
Both opportunities and challenges abound: Workflows and workforces brace for transformation, while sophisticated digital capabilities and automations are being democratized so that anyone can use — or abuse — them. As this new revolution reveals new risks, C-suites tend to look to their CISOs for answers to questions such as:
“What are the potential dangers?”
“Could this be used against us?”
“Do we have policies around this?”
“Just how worried should we be?”
“Is our organization prepared?”
Such questions are becoming common, and while they’re far from unfounded — considering what the latest AI tools are capable of — they’re difficult to answer in any meaningful way. And they neglect another fundamental truth: Security operations centers (SOCs) aren’t just guardians against AI-based risks; they also stand to gain from these new technologies as much as any other business department. In fact, they may be uniquely positioned to adopt and optimize emerging, AI-driven capabilities.
CISOs need to keep tabs on the most noteworthy and newsworthy developments in AI, like the explosion of natural language processing (NLP) and proliferation of generative AI, examining them through three different lenses:
- Bolstering offensive threat capabilities: How can emerging technologies enhance the attack methods of threat actors?
- Fortifying defensive strategies: How can they empower security operators in finding and resolving incidents?
- Distinguishing transformation from hype: Which of them can enable true transformation, and which are mere speculation?
Effectively, the purpose of this guide is threefold. First, it will provide useful definitions for the different types of AI technologies currently in play in security operations. Second, it will discuss both the positive and negative implications for the SOC, particularly when it comes to threat detection, investigation, and response (TDIR). And third, it will provide insight into the systems and solutions that lay the true groundwork for future proofed, AI-augmented security operations.
How new is artificial intelligence, really?
For the general public, it may seem like AI made its debut just recently, with little warning and a lot of buzz. But the reality is more nuanced. The technologies that constitute AI have been in development for nearly 70 years, with the first AI program publicly announced in the mid-1950s.
As a field, AI broadly refers to computer systems that are able to simulate functions associated with human cognition — for instance, analyzing information independently and using it to learn, problem-solve, and understand speech, images, and texts. Starting in the 1960s, machine learning (ML) came to the forefront as one of the most promising methods for augmenting AI capabilities, using algorithms that could ingest information from large datasets to continuously improve themselves.
Over the last decade, a subfield of ML known as “deep learning” has become the cornerstone for most of the AI technologies organizations are familiar with today. Deep learning uses multiple neural networks — collections of connected nodes that include an input layer, one or more hidden layers, and an output layer — to analyze raw, unstructured data. From this analysis, the machine can identify and distinguish features, patterns, and insights.
Some examples of deep learning include handwriting recognition, speech recognition, and image identification. Today this technology remains instrumental to modern cybersecurity through applications such as vulnerability identification, network traffic identification, and false positive tuning for security products such as IDS and IPS, as well as basic forensics.
All of the biggest breakthroughs in AI since the late 2000s — such as NLP, speech recognition, and computer vision — have been built upon the foundations laid by deep learning and neural networks. And that holds true for the latest iteration of this technology: generative AI.
Unlike other deep learning models, which specialize in classification and are thus considered “discriminative,” generative AI is, as its name implies, “generative” — it analyzes and synthesizes the inputs in its dataset to produce novel outputs. Models such as OpenAI’s DALL-E yield images from textual prompts, and GPT large language models (LLMs) translate information from training data into flexible, factual responses communicated in an intuitive, conversational way. New generative AI tools continue to refine and expand on these functions; Midjourney uses text input to create hyper-realistic and exceptionally detailed images, potentially disrupting disciplines such as visual arts and graphic design.
This has not only opened a variety of use cases for businesses — from chatbots and smart assistants, to video and image generation, to the automation of writing and coding — but the release of these models to the public has democratized the adoption of these tools. This will give CISOs pause because while there are boundless new opportunities for optimization and innovation, there is also a plethora of possible threats.
When the cat’s away, the mice will play
There’s a common refrain in cybersecurity that the relationship between adversaries and defenders is a game of cat and mouse. It’s a perpetual chase where one side gains new capabilities, forcing the other to play catch-up until they can thwart them. At that point, the roles reverse again.
Suffice it to say, the mice — or the threat actors — are already having a field day figuring out how to launch increasingly sophisticated, AI-augmented attacks. Of course, the volume of research being done on AI-enabled detection and prevention far eclipses the volume of AI-driven breach attempts at present, but as adversaries adapt and iterate on this technology, defenders must be ready to respond.
Already, AI tools are enabling bad actors to craft better phishing emails at scale. Meanwhile polymorphic malware, with its ability to escape detection systems with advanced evasion or obfuscation techniques, will likely become more sophisticated. Security leaders may recall how, in early 2020, the FBI was already cautioning that deepfake technology could pass certain biometric tests; as AI proliferates, expect more vulnerabilities to be discovered.
In a world where so many business-critical processes are automated and orchestrated “as code,” decision makers should pay careful attention to how generative AI can strengthen — and weaken — these programs. It’s not just a question of malicious code corrupting these systems; there could also be reasonable concerns around vulnerabilities being introduced by AI as it continuously automates workflows. When processes are orchestrated “as code,” they become more ephemeral and less permanent; so how can AI be held to account as it iterates and reiterates on these processes?
Adversaries are likely to exacerbate the already unsettling shift from purely opportunistic attacks to purposeful, highly targeted ones that can culminate in ransomware or outright extortion. Think about “backstopping,” where criminals will devise an entire ecosystem of nonexistent products, people, and companies to support their social engineering efforts. Now imagine that generative AI enables them to design this illusion in minutes, instead of hours or days. When it comes to network security, employees with trusted access credentials continue to be the weakest links, and emerging technologies have the potential to exploit them like never before.
For defenders that continue to solely rely on signature-based rules, generative AI is cause for concern. Of course, this technology could be used to create malicious binary code, but AI-based tools could up the ante by producing several more pieces of code that look different from the original but perform the same function. Therefore, even if defenders had stopped the first malicious binary and recorded it in their library, the rest could go unrecognized and escape detection.
These are just a few examples of the types of threats that SOCs are preparing for as user-friendly AI models become both more formidable and more accessible.
What does AI-enabled defense look like?
While AI is giving new weapons to attackers, it’s also empowering defenders. For example, the same generative AI that produces phishing emails for threat actors also enables security teams to educate their staff — and their organizations as a whole — about how to spot and guard against potential dangers. Research is also already underway to evaluate the ways in which GPT models can create synthetic data that emulates realistic attacks for fast, effective, end-to-end penetration testing.
As adversaries adopt AI for nefarious purposes, defenders can adopt the same tools to find out how they work and how to fight them. And that human element is key. While it may, in theory, be possible to automate an entire penetration test, it’s questionable whether that would be preferable to having experts conduct a red team attack, adding their nuanced knowledge to the novel AI capabilities.
It’s also essential to note that the most successful tools for cyberdefense are built on proven, well-established technologies. For example, industry-leading security information and event management (SIEM) solutions incorporate two crucial functions:
- User and entity behavior analytics (UEBA), which leverages machine learning to establish a baseline of normal activity so that threats, targeted attacks, and fraud can be found and flagged
- Security orchestration and automation response (SOAR), which can incorporate insights from deep learning systems to initiate automated defensive measures as soon as a risk is detected
Whether a threat originates with AI or not, it would still be an anomaly that deviates from the acceptable norm — and that’s why many modern SOCs already have a powerful first line of defense, even as attack methods evolve.
“Transparent” versus “opaque” AI models
All technologies that depend on deep learning algorithms — including generative AI — are trained on massive datasets and built upon complex architectures with non-linear decision boundaries. As a consequence, their outputs may be categorized as opaque, as there’s no way to know how they arrived at any given conclusion.
In some cases, there may be a degree of clarity; for instance, a tool may be purpose-built by data scientists and pre-trained on a particular dataset to solve a dedicated problem, or trained on unstructured data that nevertheless resides in an organization’s specific systems and servers. But in other cases, such as GPT-4, the outputs fundamentally lack explainability.
In contrast, SIEM technologies such as UEBA and SOAR can be considered transparent in their outputs, since they’re accountable in how they came to their conclusions:
- For transparent systems, the internal data is known, so users can understand how the outputs were derived from the inputs. UEBA is an example: An analyst will be able to explain why a specific alert was triggered.
- For opaque systems, the models are so vast there’s no way to know all the data they contain. Generative AI is like this, as are various non-UEBA solutions that analyze binary streams rather than user behavior.
As a result, there are certain risks inherent in opaque AI models that CISOs would be wise to take into account, particularly as they relate to generative AI.
Hallucination
The foundation of generative AI lies in the extensive training of LLMs on enormous amounts of content, in some cases scraped from the internet. As a content repository, the internet may contain all sorts of fallacies, biases, inaccuracies, and even intentionally corrupted information. When datasets aren’t intentionally designed, constrained, and labeled by a human expert, these flaws can emerge in unexpected ways.
Part of the problem is that when generative AI models such as GPT-4 produce new text, they deduce the next word in the sequence based on likelihood rather than factual accuracy. This contributes to a phenomenon known as hallucination, where the machine provides outputs that have the illusion of truth despite being complete nonsense. Obviously, this can have serious implications in cybersecurity if the advice of the AI is trusted without being verified. In this case, the familiar refrain, “Never trust, always verify'” takes on a whole new meaning.
Model collapse
CISOs are probably familiar with the concept of model poisoning, where a bad actor injects corrupt data into an AI’s training model. But AI systems are capable of doing this to themselves, simply by continually ingesting AI-derived content.
This is known as model collapse, and recent research demonstrates how rapidly so-called generative AI can actually degrade. At present, this process occurs inevitably as models quickly forget the original data and its distribution, and minority data characteristics such as rarities and oddities get lost. Quality control is therefore critical, and leaders should be concerned about cascading failures if outputs aren’t checked regularly.
Non-compliance
When opaque models ingest data, what’s to stop them from using sensitive or proprietary information? This should be a concern for CISOs contemplating generative AI capabilities, because once that data has disappeared into the training model, it’s gone — and it’s conceivable that there could be regulatory liabilities depending on the type of data that has been intentionally or unintentionally shared.
Machine learning is still driving detection
Generative AI may be grabbing headlines, and justifiably so, but it’s apparent that the latest, greatest strides in NLP aren’t really helping cybersecurity leaders with their critical threat detection, investigation, response, and remediation capabilities. ML tools still rule when it comes to detection, while automation and traditional human-led forensics are crucial for remediation.
SIEM capabilities such as UEBA use machine learning to establish a baseline of normal behavior for users, devices, and other entities in an organization’s environment, and alert the SOC when there are deviations. Although attack techniques, zero-day vulnerabilities, and attackers’ tools and tactics change, the framework outlining the impact within an environment remains a constant. In this context, UEBA persists as the most effective way to detect them. Meanwhile, SOAR remains a reliable method for SOCs to streamline and automate their response. It’s also important to note that the processing power of cloud-native SIEM platforms is ideal for scaling UEBA workloads.
At the end of the day, multiple factors come together to holistically enhance an organization’s security posture. ML optimizes and adapts to detect behavior. Automated tools assist with response and remediation. And human analysts continue to be essential in driving the entire process with their unique ability to provide the oversight and context that computer systems still lack.
Assessing the impact of AI on the SOC
Although threat detection and remediation are still within the domain of deep learning and automation tools, there’s plenty of potential for generative AI to support the investigation and response aspects of aforementioned TDIR processes. An AI tool capable of immediately articulating threats and offering recommendations for initial actions could be incredibly helpful when an alert is generated. And the automatic, dynamic creation of playbooks could help accelerate investigations and responses in the future.
Looking further, there are other possible use cases for this type of AI that would provide undeniable value to CISOs and security teams:
- One of the most compelling is the utilization of advanced NLP to simplify code, such as SQL statements: these are the queries that allow users to search, structure, summarize, alter, and update databases. Rather than adhering to rigorously technical prompts, a security analyst would be able to retrieve logs and reports using common, everyday language.
- Taking the query statements a step further, AI tools that use sophisticated NLP may be able to glean greater insights from dashboards and visualizations. Instead of seeing SOC metrics on MTTR and case type as a single snapshot in time, AI has the potential to reveal month over month changes and provide intel on the factors driving those changes.
- Another impressive capability would be proactive threat hunting. While today’s TDIR tools analyze network activity and user behavior in real time, tomorrow’s AI may be able to use a combination of historical data and predictive analytics to anticipate vulnerabilities and intrusions long before they can materialize.
While such solutions don’t necessarily change the underlying technologies that drive TDIR, they do streamline and automate processes for security operations. By freeing security practitioners from tedious, technical, and time-consuming tasks, teams can be more aware of alerts and incidents holistically, and bring their critical thinking and judgment to bear where it matters most.
It goes without saying that there’s currently a vast amount of investment in AI — particularly generative AI, which continues to grow in sophistication. It’s not unrealistic to imagine a near-future scenario where AI is able to offer novel or predictive insights to support security analysts in their TDIR workflows, connecting dots and delivering observations that may have taken hours for a human to uncover.
For now, it’s important for business leaders to continue managing their expectations when it comes to the latest wave of AI tools. There are still difficulties in ensuring the accuracy of AI-derived content, and ongoing controversy around the ethical use of AI-derived media. There’s also a growing awareness of the practical limitations of AI in its current form as the novelty of GPT-3 and GPT-4 starts to wear off.
Laying a foundation for a changing future
As new products — and new promises — hit the market, CISOs should always mind their gaps. In terms of resources, capabilities, and vulnerabilities, what’s currently missing from the SOC? Can AI credibly fill these voids, especially for repetitive tasks? Vendors and their products must be vetted conscientiously to guarantee that they’re adding real value. Be wary of AI tools that make sweeping, general proclamations, and focus on those that solve genuine, specific problems; and be wary of companies that lead with AI, rather than focusing on cybersecurity and the practical application of AI to potential use cases.
Many security professionals will remember the proliferation of vendors making outsized — and often outlandish — claims about their products’ AI capabilities back when deep learning went mainstream. A similar proliferation also occurred around zero trust tools when “never trust, always verify” became an industry best practice. Generative AI will likely garner a great deal of hype as well, and decision makers will need to perform their due diligence to discern what’s a valid, valuable solution, and what’s just opportunistic marketing.
CISOs are also people leaders, and it’s imperative to think about how the integration of AI into the SOC can facilitate professional development beyond productivity boosts, as well as grow cybersecurity staff in their knowledge, capabilities, and careers. Disciplines such as data science, statistical modeling, and the emerging field of prompt engineering are going to become increasingly necessary for security professionals in the future. Helping teams gain a strong foundation in these areas now offers them a valuable advantage, which will benefit both their growth and the overall success of the organization.
Ultimately, the goal of any great leader is to be a great simplifier. Complexity is the enemy of security. Looking across people, processes, and technology, what areas present opportunities for improvement? What can be accelerated? And how do current and nascent AI use cases support this? In an industry in the throes of change, it’s these simple, yet powerful, priorities that can keep CISOs and SOCs on track.
Related Articles
Join The GBI Impact Community
Sign up to make an impact and hear about our upcoming events
By registering anywhere on the site, you agree with our terms and privacy policy