What wasting data engineering talent really costs you
Data engineers spend almost half their time maintaining data pipelines. The total average cost? $520,000 per year, according to new research.
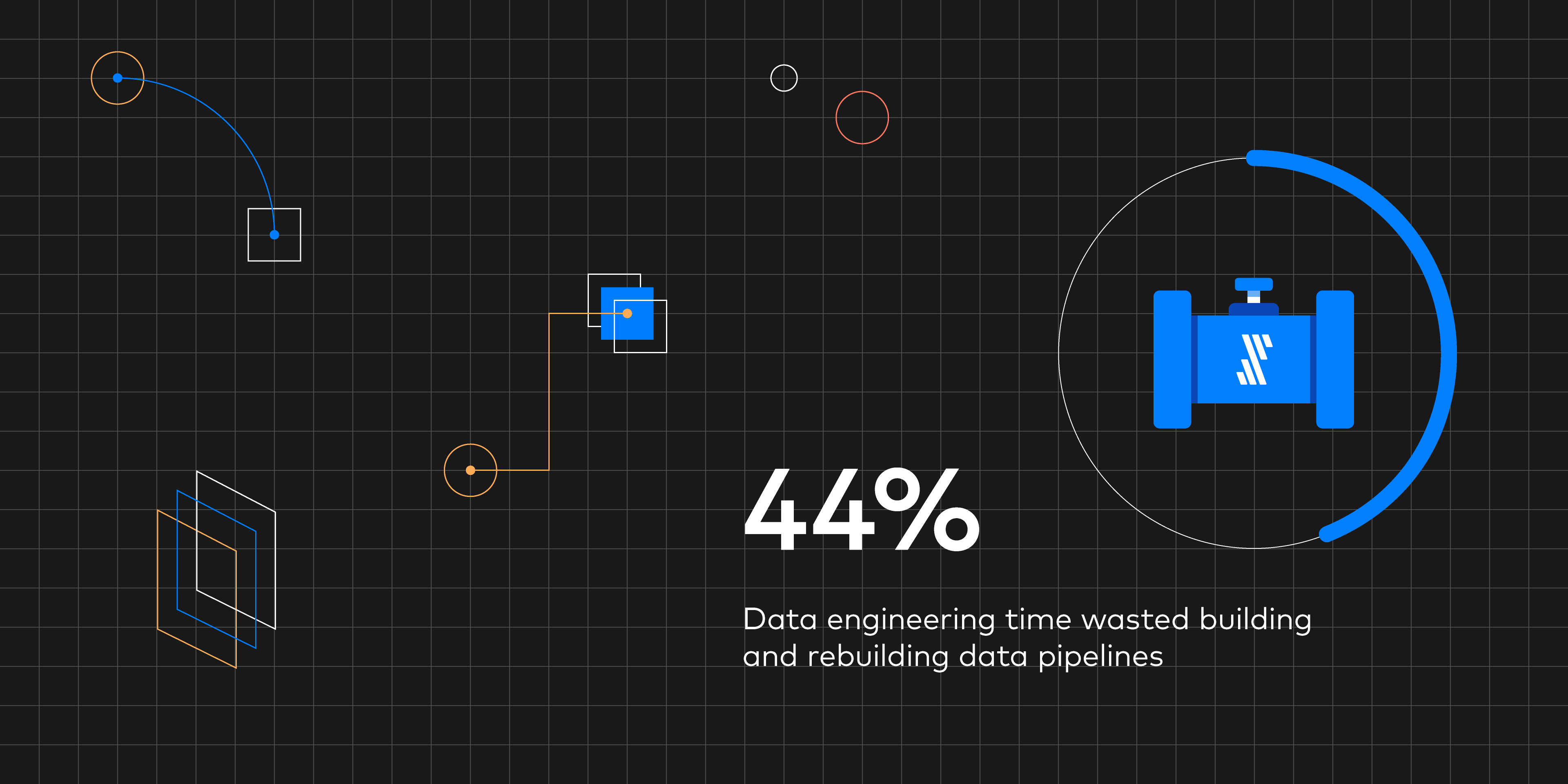
Building a modern data stack from scratch is complex. Using traditional APIs and legacy data integration tools is tedious and expensive. Collecting, cleaning, sorting and analyzing data on an enterprise scale across dozens of internal and third-party sources takes an entire team of data professionals working around the clock, constantly maintaining and updating these complex pipelines.
But a functioning data stack is a critical need. Data drives business, giving companies real-time insight into market conditions, dynamic supply chains and unpredictable customer expectations so they can make quick, accurate decisions in the moment.
Organizations that are able to radically transform their approach to integrating data by embracing an automated, efficient approach will save valuable engineering time and create critical insights that will result in major business impact in a competitive market.
Unfortunately, many companies are letting data infrastructure inefficiencies sap their engineering resources. According to a new report by Wakefield Research, the average data engineer spends 44 percent of their time maintaining data pipelines – which costs $520,000 per year.
It’s no surprise that, according to the same report from Wakefield, nearly three out of four data engineers feel that their team’s time and talent are being wasted by having to manage these data pipelines manually.
Can you blame them? Refocusing their responsibilities away from manual maintenance to data analytics would go a long way toward improving morale and retention.
So, why ask a highly-trained data engineer to spend their time twisting knobs and pulling levers? Shouldn’t they be, you know, actually working with data?
The agony of a manual data pipeline
Unfortunately, legacy data ingestion tools are ill suited for today’s enterprise data needs. First, it can take a data engineer weeks or even months to build a single connector. Multiply that by the number of connectors needed — often dozens or even hundreds within a single company, depending on the data environment — and you’re talking about a multi-year project just to get everything fed into your data warehouse.
Once configured, legacy tools may require engineers to manually set up hundreds or thousands of schemas and tables one at a time to ensure the data is landed in the desired format. There’s also the issue of post-build maintenance: what happens after you’ve created a custom data pipeline. Your new connector can pull your data from a given source, but now you have to continually maintain the code and system infrastructure. Every time your source issues an update to its API or data structures, you’ll need to accommodate new API endpoints and supported fields, resulting in updating your pipeline extract scripts. And that takes resources away from the data engineering team.
Data integration as a service
An alternative to the DIY route is a managed data integration service to build, manage and update data pipelines. This allows companies to automate the process of integrating data into their data warehouse, eliminating ongoing maintenance and all the hassles of updating pipelines when APIs or data structures change, and giving data engineers valuable time back.
“My job is to make sure everyone has the information they need to create efficiencies, deliver superior experiences for customers and optimize their efforts,” said Daniel Deng, a data architect at mortgage broker Lendi. “I need to make it as easy as possible to work with and analyze the data from various data sources. The quicker we get the data, the quicker we get the insights that the business needs for making a decision. Then the quicker our business operates and evolves.”
The impact of automating the data pipeline was even more significant for Mark Sussman, head of data analytics for ItsaCheckmate. He was able to stand up an entire data analytics framework in just a few months with minimal engineering help. “I can honestly say that we are now a data-driven company,” Mark said. “Information is far more readily available to deal with any customer problem quickly and efficiently, and we can focus on optimizing customer experiences. But, most of all, we’re focused on growth.”
This aligns with Wakefield’s findings. According to the report, 69 percent of data and analytics leaders said business outcomes would improve if their teams were able to spend less time on manual pipeline management.
What you could be doing
So, what exactly would data engineers do with 44 percent more time? We’re glad you asked.
- Build new, advanced models and analytics
Not having to manually ingest the data and manage data pipelines allows data engineers to do what they do best. This includes building new models and analytics.
For example, data engineers at Canva pull marketing and sales analytics from dozens of engagement platforms in the cloud to the company’s data platform managed by Snowflake. Insights derived through the company’s business intelligence tools, Looker and Mode Analytics, allow Canva’s marketers to make necessary tweaks and optimize results. Automatically pulling in data from across the marketing stack — social, email and digital advertising — enables comparison of campaign performance across Canva and third-party platforms while saving Canva over $200,000 per year in engineering costs. They can then model the information to provide a complete, 360-degree view of the customer.
- Democratize access to data and build a culture of data literacy
Aman Malhotra, the head of growth and analytics at Super Dispatch, has seen the demand for data explode since automating the company’s data pipelines and providing team insights to help reach their specific goals. She is inundated with data requests from her colleagues across the company (in a good way, because she can easily address these requests). Instead of relying on gut feelings or overloading the data analytics team with requests, Super Dispatch employees can dive into the data themselves in whatever way they see fit. The ease of data access has made everyone want to get their hands on actionable data that they can use to make the right decision at the right time leading to positive, measurable results.
Compare that to what the research found about the current state of affairs at most companies: 76 percent of data engineers report that it takes them days or a week to prepare data for decisions that affect revenue.
- Build internal apps to generate business value
Data engineers can also spend more time developing internal applications that automate and streamline internal processes. Daydream, an early-stage startup in the finance space, automates much of its data pipeline, which frees up its data team to focus on building out the company’s core product. This ability to refocus engineering resources allowed the company to speed its time to market by a full month — a huge advantage in a crowded, up-and-coming market.
- Always look for opportunities to automate
With data integration problems solved, data engineers can focus on other tools in the data stack to get the most value out of them. For example, Australian online retailer Sleeping Duck pulls in data from 24 different data sources – from Google Analytics to Xero, the company’s accounting platform – to make critical business decisions across marketing, sales and customer success. For example, building and managing these data ingestion pipelines in-house would have doubled the total time needed to understand what marketing campaigns work versus not to optimize spend. Automating their data integration process has given them the time and resources to gain valuable insights and transform the way it does business and ultimately deliver a better customer experience.
How to free up your time for more productive uses
Data engineering time is scarce, and building a modern data stack from scratch is tough. It costs the average company $520,000 per year to manually wrangle data. That time and money is better spent on actually working with the data to derive powerful business insights that develop the core product, streamline internal processes and improve the customer experience. Only then can you optimize your modern data pipeline and build a data-driven culture at your company.
Download “The State of Data Management Report” for an in-depth look at the survey results of 300 data and analytics leaders by Wakefield Research and Fivetran.
Related Articles
Join The GBI Impact Community
Sign up to make an impact and hear about our upcoming events
By registering anywhere on the site, you agree with our terms and privacy policy