Why Your AI Strategy Is Only as Good as the Data Behind It
For enterprises to reap the disruptive benefits of far-ranging AI capabilities, they must first unlock the data piece. Specifically, a key to ensuring the successful implementation of next-gen technology in business, experts say, is refining the processes around how to obtain data, how to house it efficiently, governing who can access it, and how it should be used to drive desired outcomes. In essence, the commercial impact of AI hinges in large part on how proficiently a business can work with the “raw material.”
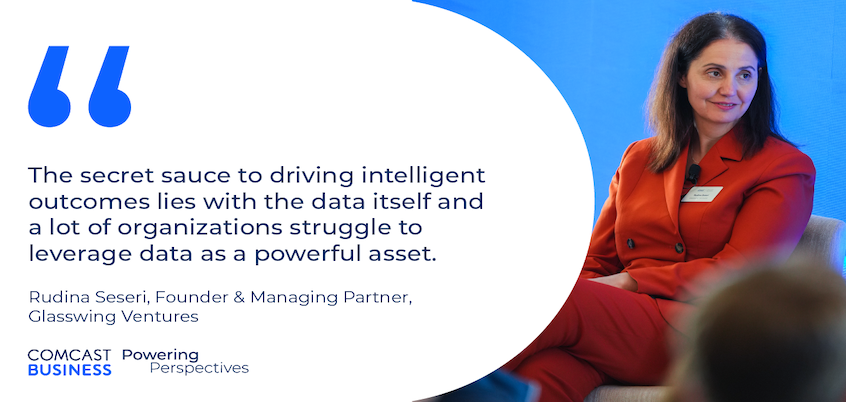
“The secret sauce to driving intelligent outcomes lies with the data itself,” said Rudina Seseri, Co-Founder and Managing Partner of Glasswing Ventures, “and a lot of organizations struggle to leverage data as a powerful asset.” According to research by Morning Consult and IBM, “too much data complexity” is a frequent barrier to successful AI adoption for 25% of enterprises.
“Enterprises should not assume that simply because they have the data, and they have ML models, strong outcomes will naturally occur,” said Seseri, who was among other AI experts speaking to CIOs and other business leaders during a series of panel discussions hosted by Comcast Business at THE PLAYERS® Championship. “Managing the cost, complexity and preparation of the data that enterprises feed into their ML model is just as important and deterministic as the model itself.”
Data governance requires a rigorous approach, mainly—though not only— because high-quality data is required for AI systems to reliably interpret huge datasets and derive accurate and meaningful insights. And the onus is on the enterprise to track the chain of custody for data that is fed into AI models, the panelists said.
Rick Rioboli, EVP and CTO of Comcast, shared during the panel discussions that Comcast has successfully created what he calls a “data fabric” that consolidates data hubs in various locations to create “one big elastic search capability” so that developers have a place to turn to when looking for data.
The efforts have alleviated the previous pain point of “having data all over the place,” by creating a “semantic layer that allows us to start talking about the same data with the same descriptions,” Rioboli explained.
As more firms uncover more processes they can improve with AI, creating more data infrastructure models will present unique opportunities for both disruption and automation. For example, there are AI-based self-service feature platforms that can automate the processing of data before it can be fed into a model. One such platform purports to drastically cut down the data prepping and coding work that would typically take dozens of data scientists and data engineers months to do—to just one day.
In the end, companies need to focus on aligning “clean” data with the right AI strategy to unlock the true potential of emerging technologies and drive business outcomes.
Check out additional perspectives from our conversations at THE PLAYERS® Championship.
Related Articles
Join The GBI Impact Community
Sign up to make an impact and hear about our upcoming events
By registering anywhere on the site, you agree with our terms and privacy policy